Screening programs are crucial for early detection of breast cancer, and mammography remains the medical imaging standard. Traditional mammography provides 2D images of breast tissue, but when combined with digital breast tomosynthesis (DBT), or “3D mammography”, it becomes more likely to detect cancer, especially in dense breast tissue.
These 3D DBT images are built from series of two-dimensional images and are typically 10x or 100x their 2D counterparts in total size, which doubles interpretation time for radiologists. Artificial intelligence might be the solution. Researchers have been developing and using AI software that can routinely and efficiently evaluate the presence of cancer in large image datasets. AI software can also identify patterns and abnormalities that human radiologists might miss.
In a recent study published in IEEE Transactions On Medical Imaging, researchers at NYU Langone Health have developed an AI-based 3D imaging model, called 3D-GMIC, that greatly improves computational efficiency without reducing accuracy.
“We save computation by selecting certain areas of the image for deeper analysis.”
Krzysztof J. Geras, PhD
According to Krzysztof J. Geras, PhD, a machine learning expert and senior researcher on the study, “In this AI model, we were trying to intuitively reproduce the way radiologists look at images. We save computation by selecting certain areas of the image for deeper analysis.”
Performance Through Localization
The 3D-GMIC model builds on a labeled dataset of breast images that took NYU Langone researchers years to compile. The model, developed by Jungkyu Park—a doctoral student in Dr. Geras’ group and first author of the study—and colleagues, was trained with image-level labels only. This included elements like whether or not a patient has cancer, as it is challenging to manually build a dataset of localized, labeled regions of cancer within large 3D images.
3D-GMIC learns to highlight the important regions, enabling it to learn from the data without localization labels. “It is actually pretty remarkable that you can localize these cancers without the localization labels,” says Dr. Geras.
In terms of image analysis, DBT images are comprised of 70 slices on average, each containing a few million pixels. “With such large images, humans would never look at the entire image all at once,” says Geras.
3D-GMIC predicts the malignancy of lesions and localizes them in 3D images. First, it uses a global model to identify “patches” in the image that require more attention. “We then look at these patches with a local model, a higher capacity network,” says Dr. Geras.
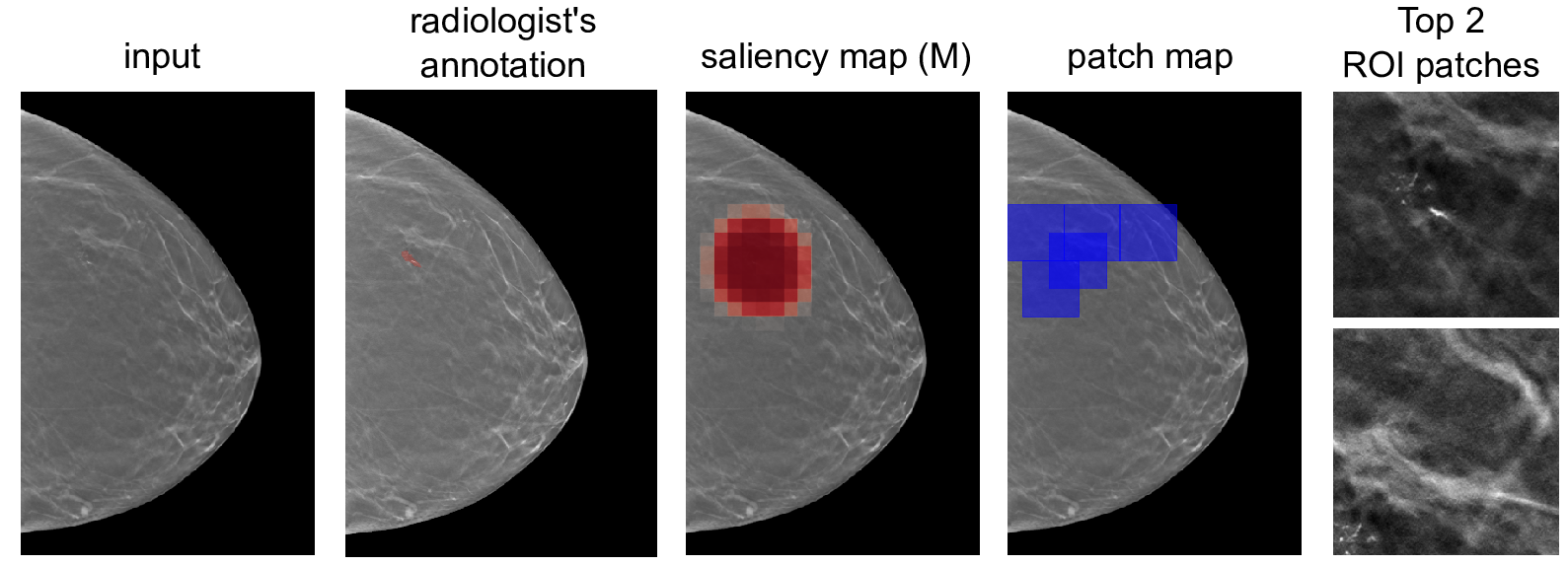
By focusing on specific patches, researchers found that 3D-GMIC uses 78 to 90 percent less GPU memory, and 91 to 96 percent less computation than existing AI models. Despite this significant reduction, 3D-GMIC still showed comparable performance to earlier models, demonstrating that it successfully classified large 3D images.
Finding Practical Utility
A promising feature of this study is that the 3D-GMIC model generalized well to an external image dataset from Duke University Hospital. With AI-based medical imaging, it’s challenging to build models that work across different locations. “The fact that this is working across different sources of data shows this method is robust, which is an important condition for the practical utility of these models,” says Dr. Geras.
“When we give expert breast imagers access to models such as this, we improve patient care. We find more cancers, and we find different cancers than the ones that the radiologists find.”
Laura Heacock, MD
According to Laura Heacock, MD, an associate professor of radiology at NYU Langone, they are continuing to test the model in clinical settings. “We’ve found that when we give expert breast imagers access to models such as this, we improve patient care,” says Dr. Heacock. “We find more cancers, and we find different cancers than the ones that the radiologists find.”
This study is part of a long-term research project on AI for all modalities of breast imaging, including DBT, ultrasound, and MRI. These imaging modalities all “say something different about the tissue of the patient,” says Dr. Geras. Developing a neural network that could look at mammography, ultrasounds, and MRIs has the potential to lead to more accurate diagnoses.